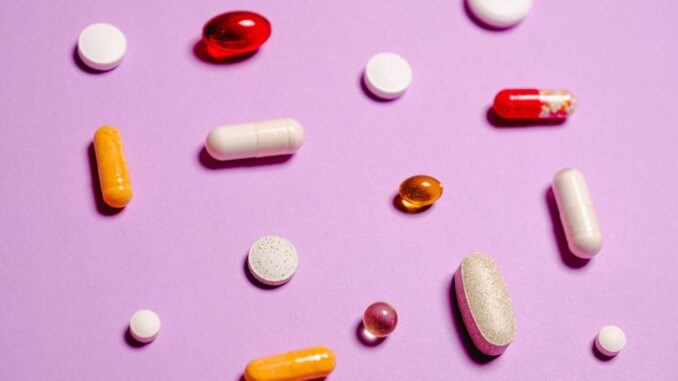
The Expanding Frontier of Data Utilization in Addiction Treatment: Navigating Complexity and Enhancing Precision
Many thanks to our sponsor Maggie who helped us prepare this research report.
Abstract
Addiction treatment is undergoing a paradigm shift, moving towards data-driven approaches to enhance efficacy and personalize care. This report examines the multifaceted role of data in revolutionizing addiction treatment. It explores various data types relevant to addiction, including patient demographics, treatment histories, biological markers, and socio-environmental factors, emphasizing the importance of integrating diverse data sources for a holistic understanding. We delve into advanced methodologies for data analysis, such as machine learning and causal inference, and their potential to identify predictive biomarkers, optimize treatment strategies, and understand complex interactions. Furthermore, the report addresses critical ethical considerations, including data privacy, algorithmic bias, and informed consent, proposing robust frameworks for responsible data utilization. Finally, we discuss best practices for data security, interoperability, and collaboration to facilitate the translation of data-driven insights into tangible improvements in addiction treatment outcomes. We will look at the limitations and possible improvements in existing data collection and use, and how to improve outcomes for patients and treatment centers.
Many thanks to our sponsor Maggie who helped us prepare this research report.
1. Introduction
Addiction, a chronic relapsing brain disease, presents a significant global health challenge. Traditional approaches to addiction treatment have often relied on generalized protocols, overlooking the individual heterogeneity of patients and the complex interplay of biological, psychological, and social factors contributing to addiction. The limitations of these approaches have fueled the need for more personalized and effective interventions. The increasing availability of vast datasets, coupled with advancements in data analytics, offers a powerful opportunity to transform addiction treatment.
This research report explores the evolving landscape of data utilization in addiction treatment. It examines the types of data that can be leveraged, the methods for analyzing this data, the ethical considerations that must be addressed, and the potential benefits of data-driven insights for improving patient outcomes. The report aims to provide a comprehensive overview of the opportunities and challenges in this rapidly developing field, offering insights relevant to researchers, clinicians, policymakers, and technology developers.
Many thanks to our sponsor Maggie who helped us prepare this research report.
2. Data Types in Addiction Treatment: A Comprehensive Overview
The effectiveness of data-driven approaches hinges on the availability of comprehensive and high-quality data. In the context of addiction treatment, a wide range of data types can be collected and integrated to provide a holistic view of the patient and their recovery journey. These data types can be broadly categorized as follows:
-
Patient Demographics and Clinical Characteristics: This category includes fundamental information about the patient, such as age, gender, ethnicity, socioeconomic status, education level, and employment status. It also encompasses clinical characteristics, including addiction type (e.g., opioid, alcohol, stimulant), severity of addiction (assessed through standardized scales like the Addiction Severity Index (ASI) [1] or the Diagnostic and Statistical Manual of Mental Disorders (DSM) criteria [2]), co-occurring mental health disorders (e.g., depression, anxiety, PTSD), and medical history (e.g., chronic pain, liver disease). A robust understanding of these baseline characteristics is critical for risk stratification and tailoring treatment plans.
-
Treatment History: A detailed record of the patient’s past treatment experiences is invaluable. This includes information on previous treatment modalities (e.g., medication-assisted treatment (MAT), cognitive behavioral therapy (CBT), motivational interviewing (MI)), treatment duration, adherence to treatment, and treatment outcomes. Analyzing treatment history can help identify patterns of treatment response, predict future outcomes, and avoid repeating ineffective strategies.
-
Biological and Genetic Data: Advances in genomics and biomarker research offer the potential to identify biological markers that predict addiction risk, treatment response, and relapse vulnerability. Genetic data, including polymorphisms in genes related to reward pathways, neurotransmitter systems, and drug metabolism, can provide insights into individual susceptibility to addiction [3]. Biomarkers, such as levels of stress hormones (e.g., cortisol) or inflammatory markers (e.g., C-reactive protein), can reflect the physiological impact of addiction and treatment [4]. Integrating biological and genetic data into clinical practice is an emerging area with significant promise.
-
Socio-Environmental Data: Addiction is strongly influenced by social and environmental factors, including social support networks, living environment, exposure to trauma, and access to resources. Data on these factors can be collected through surveys, interviews, and social network analysis. Understanding the patient’s social context is essential for developing comprehensive treatment plans that address the social determinants of addiction.
-
Real-World Data (RWD) and Patient-Generated Health Data (PGHD): RWD includes data collected outside of traditional clinical settings, such as data from electronic health records (EHRs), claims databases, wearable sensors, and mobile apps. PGHD refers to data created by patients themselves, such as self-reported symptoms, mood ratings, and activity levels. These data sources provide valuable insights into the patient’s daily experiences, treatment adherence, and overall well-being [5]. Utilizing RWD and PGHD can enhance the ecological validity of addiction treatment research and inform more personalized interventions.
Many thanks to our sponsor Maggie who helped us prepare this research report.
3. Data Analysis Methods: Unlocking Insights from Complex Data
The wealth of data available in addiction treatment requires sophisticated analytical methods to extract meaningful insights. Several data analysis techniques are particularly relevant:
-
Statistical Analysis: Traditional statistical methods, such as regression analysis, ANOVA, and t-tests, are foundational for analyzing group differences, identifying predictors of treatment outcomes, and evaluating the effectiveness of interventions. These methods are well-established and widely used in addiction research.
-
Machine Learning (ML): ML algorithms can identify complex patterns and relationships in data that are not readily apparent through traditional statistical methods. ML techniques, such as supervised learning (e.g., classification, regression) and unsupervised learning (e.g., clustering, dimensionality reduction), can be used to predict treatment outcomes, identify patient subgroups, and personalize treatment plans [6]. For example, ML algorithms can predict the likelihood of relapse based on patient demographics, treatment history, and biological markers. ML can also be used to create individualized treatment plans based on patient data and outcomes.
-
Causal Inference: Establishing causal relationships between interventions and outcomes is crucial for informing clinical decision-making. Causal inference methods, such as propensity score matching, instrumental variables, and mediation analysis, can help disentangle the effects of treatments from confounding factors [7]. These methods are particularly important in observational studies where randomization is not possible.
-
Network Analysis: Addiction is influenced by complex interactions between individuals, social networks, and environmental factors. Network analysis techniques can be used to map these interactions and identify key influencers and vulnerabilities. For example, network analysis can reveal how social support networks impact treatment adherence and relapse risk [8].
-
Time Series Analysis: Longitudinal data, such as data collected from wearable sensors or mobile apps, requires time series analysis methods to capture temporal patterns and trends. Time series analysis can be used to identify early warning signs of relapse or to assess the impact of interventions over time.
-
Natural Language Processing (NLP): NLP techniques can extract information from unstructured text data, such as clinical notes and patient narratives. NLP can be used to identify key themes, assess sentiment, and track changes in patient well-being over time [9].
Choosing the appropriate data analysis method depends on the research question, the type of data available, and the desired level of inference. It is often beneficial to combine multiple methods to gain a more comprehensive understanding of the data.
Many thanks to our sponsor Maggie who helped us prepare this research report.
4. Ethical Considerations: Navigating the Moral Landscape of Data-Driven Addiction Treatment
The use of data in addiction treatment raises significant ethical considerations that must be carefully addressed. These considerations include:
-
Data Privacy and Confidentiality: Addiction is a highly stigmatized condition, and patients may be reluctant to share sensitive information if they fear it will be disclosed to others. It is crucial to protect patient privacy and confidentiality by implementing robust data security measures and adhering to ethical guidelines, such as the Health Insurance Portability and Accountability Act (HIPAA) [10]. De-identification and anonymization techniques can be used to reduce the risk of data breaches and protect patient identities.
-
Informed Consent: Patients must be fully informed about how their data will be used, who will have access to it, and the potential risks and benefits of data sharing. Informed consent should be obtained prior to collecting and using patient data for research or clinical purposes. The consent process should be transparent and understandable, ensuring that patients have the autonomy to make informed decisions about their data.
-
Algorithmic Bias: ML algorithms can perpetuate and amplify existing biases in data, leading to unfair or discriminatory outcomes. It is crucial to identify and mitigate algorithmic bias by using diverse and representative datasets, carefully evaluating algorithm performance across different subgroups, and implementing fairness-aware algorithms [11]. For example, if an algorithm is trained primarily on data from male patients, it may not accurately predict outcomes for female patients.
-
Data Ownership and Control: Patients should have control over their data and the ability to access, modify, and delete their information. Data ownership policies should be transparent and equitable, ensuring that patients have a say in how their data is used.
-
Data Security: Protecting data from cybersecurity attacks or data breaches is important. Implementing robust cybersecurity measures, such as encryption, firewalls, and access controls, is essential for safeguarding patient data. Data security policies should be regularly reviewed and updated to address evolving threats.
-
Transparency and Accountability: Data-driven decision-making should be transparent and accountable. The rationale behind algorithms and treatment recommendations should be clearly explained to patients and clinicians. Mechanisms should be in place to address errors and biases in data-driven systems.
Addressing these ethical considerations is essential for building trust and ensuring that data is used responsibly and ethically in addiction treatment.
Many thanks to our sponsor Maggie who helped us prepare this research report.
5. Best Practices for Data Security and Privacy
Given the sensitive nature of patient data in addiction treatment, implementing robust security and privacy measures is paramount. Here are best practices for protecting patient information:
-
Data Encryption: Employ strong encryption methods to protect data both in transit and at rest. This includes encrypting data stored on servers, databases, and portable devices, as well as encrypting data transmitted over networks.
-
Access Controls: Implement strict access controls to limit access to patient data to authorized personnel only. Use role-based access control (RBAC) to assign permissions based on job responsibilities. Regularly review and update access controls to ensure they remain appropriate.
-
Data Anonymization and De-identification: Use data anonymization and de-identification techniques to remove or mask personally identifiable information (PII) from datasets. This can reduce the risk of data breaches and protect patient identities while still allowing for valuable research and analysis.
-
Data Governance Policies: Establish clear data governance policies that define how data should be collected, stored, used, and shared. These policies should address issues such as data quality, data retention, data access, and data security. Regularly review and update data governance policies to ensure they align with evolving regulations and best practices.
-
Employee Training: Provide comprehensive training to all employees on data security and privacy best practices. This training should cover topics such as data protection, password security, phishing awareness, and incident response. Regularly reinforce training through ongoing education and awareness campaigns.
-
Data Breach Response Plan: Develop a comprehensive data breach response plan that outlines the steps to be taken in the event of a data breach. This plan should include procedures for identifying, containing, and mitigating the breach, as well as notifying affected individuals and regulatory agencies.
-
Regular Security Audits: Conduct regular security audits to identify vulnerabilities and weaknesses in data security systems. These audits should be performed by qualified security professionals and should cover all aspects of data security, including network security, application security, and physical security.
-
Compliance with Regulations: Ensure compliance with all applicable data security and privacy regulations, such as HIPAA, GDPR, and state-specific laws. Stay up-to-date on regulatory changes and adapt data security practices accordingly.
-
Secure Data Sharing: Establish secure data sharing agreements with third-party partners and collaborators. These agreements should outline the responsibilities of each party for protecting patient data and should include provisions for data security, data privacy, and data governance.
By implementing these best practices, addiction treatment providers can significantly reduce the risk of data breaches and protect patient privacy.
Many thanks to our sponsor Maggie who helped us prepare this research report.
6. Applications of Data-Driven Insights in Addiction Treatment
The insights derived from data analysis can be applied to various aspects of addiction treatment, leading to improved outcomes and more efficient resource allocation. Some key applications include:
-
Personalized Treatment Planning: Data-driven insights can be used to develop personalized treatment plans that are tailored to the individual needs of each patient. By analyzing patient demographics, treatment history, biological markers, and socio-environmental factors, clinicians can identify the most effective treatment modalities for each patient [12]. Personalized treatment planning can lead to improved treatment adherence, reduced relapse rates, and better overall outcomes.
-
Predictive Analytics for Relapse Prevention: ML algorithms can be used to predict the likelihood of relapse based on patient data. This allows clinicians to identify patients who are at high risk of relapse and to implement preventive interventions, such as relapse prevention therapy or medication management. Predictive analytics can help to reduce relapse rates and improve long-term recovery outcomes [13].
-
Resource Allocation and Optimization: Data-driven insights can be used to optimize the allocation of resources in addiction treatment centers. By analyzing data on patient flow, treatment utilization, and outcomes, administrators can identify areas where resources are being underutilized or overutilized. This allows them to make more informed decisions about staffing, program development, and facility management [14].
-
Outcome Monitoring and Evaluation: Data can be used to monitor treatment outcomes and evaluate the effectiveness of different treatment modalities. By tracking patient progress over time, clinicians can identify areas where treatment is working well and areas where it needs to be improved. Outcome monitoring and evaluation can help to ensure that treatment programs are delivering the best possible results.
-
Early Intervention and Prevention: Data can be used to identify individuals who are at high risk of developing addiction. By analyzing data on risk factors, such as family history of addiction, exposure to trauma, and social isolation, public health officials can implement targeted prevention programs. Early intervention and prevention can help to reduce the incidence of addiction and improve public health outcomes.
-
Improving Treatment Adherence: One of the biggest challenges in addiction treatment is adherence. By combining data from various sources, including electronic health records, medication adherence monitoring, and patient-reported data, it’s possible to identify patients who are struggling to adhere to their treatment plans. Personalized interventions can then be implemented to support these patients, such as medication reminders, counseling sessions, or peer support groups.
-
Understanding Treatment Heterogeneity: While broad treatment guidelines exist, not all patients respond the same way to the same treatment. Data analysis can help identify subgroups of patients who benefit most from specific treatments, leading to a more targeted and effective approach. This could involve identifying biomarkers, genetic factors, or demographic characteristics that predict treatment response.
Many thanks to our sponsor Maggie who helped us prepare this research report.
7. Challenges and Future Directions
Despite the significant potential of data utilization in addiction treatment, several challenges remain. These challenges include:
-
Data Silos and Interoperability: Data is often fragmented across different systems and organizations, making it difficult to integrate and analyze. Improving data interoperability is crucial for enabling data-driven insights. This can be achieved through the adoption of standardized data formats, data exchange protocols, and data governance policies [15].
-
Data Quality and Completeness: The accuracy and completeness of data are essential for generating reliable insights. However, data in addiction treatment settings is often incomplete or inaccurate due to factors such as manual data entry, inconsistent data collection practices, and missing data. Improving data quality requires implementing data validation procedures, providing training to data entry personnel, and using automated data collection tools.
-
Lack of Data Science Expertise: Data analysis requires specialized skills and expertise. However, many addiction treatment centers lack the resources to hire data scientists or to train existing staff in data analysis techniques. Addressing this challenge requires investing in data science education and training programs, as well as fostering collaborations between addiction treatment centers and data science experts.
-
Resistance to Change: Implementing data-driven approaches requires a change in culture and mindset. Some clinicians and administrators may be resistant to adopting new technologies or data analysis methods. Overcoming this resistance requires providing education and training on the benefits of data-driven approaches, as well as involving stakeholders in the implementation process.
Looking forward, several key areas for future development include:
-
Development of Standardized Data Sets: The creation of standardized data sets for addiction research and treatment will facilitate data sharing and collaboration, leading to more robust and generalizable findings.
-
Integration of Artificial Intelligence (AI): AI has the potential to revolutionize addiction treatment by automating tasks, improving decision-making, and personalizing interventions. However, the use of AI in addiction treatment must be carefully considered to ensure that it is ethical, transparent, and accountable [16].
-
Expansion of Real-World Data Collection: Expanding the collection of RWD and PGHD will provide a more comprehensive and ecologically valid picture of the patient’s recovery journey. This will require the development of user-friendly data collection tools and the implementation of data security and privacy measures.
-
Focus on Implementation Science: Implementation science is the study of methods to promote the adoption and implementation of evidence-based practices. Applying implementation science principles to data-driven addiction treatment can help to ensure that new technologies and methods are effectively integrated into clinical practice [17].
By addressing these challenges and pursuing these future directions, the field of data utilization in addiction treatment can realize its full potential to improve patient outcomes and transform the delivery of care.
Many thanks to our sponsor Maggie who helped us prepare this research report.
8. Conclusion
The integration of data into addiction treatment holds immense promise for improving outcomes, personalizing care, and optimizing resource allocation. By leveraging diverse data sources, employing advanced analytical methods, and adhering to ethical principles, we can unlock the potential of data to transform the landscape of addiction treatment. Overcoming the challenges related to data interoperability, quality, and expertise, while embracing future developments in AI and implementation science, will pave the way for a more effective, equitable, and data-driven approach to addiction recovery. As technology continues to advance and data becomes more readily available, the field of addiction treatment must continue to adapt and evolve to leverage the power of data to improve the lives of individuals and communities affected by addiction. It is also important to continue to develop new treatments and methods, not just rely on existing ones, to continue to improve treatment success.
Many thanks to our sponsor Maggie who helped us prepare this research report.
References
[1] McLellan, A. T., Kushner, H., Metzger, D., Peters, R., Smith, I., Grissom, G., … & Arndt, I. O. (1992). The Fifth Edition of the Addiction Severity Index. Journal of Substance Abuse Treatment, 9(3), 199-213.
[2] American Psychiatric Association. (2013). Diagnostic and statistical manual of mental disorders (5th ed.). Washington, DC: Author.
[3] Uhl, G. R., Koob, G. F., Cable, J., & Li, T. K. (2008). The neurobiology of addiction: update 2007. The Journal of addictive diseases, 27(1), 1-17.
[4] Sinha, R. (2001). How does stress increase risk of drug abuse and relapse?. Psychopharmacology, 158(4), 343-359.
[5] Patel, S., Park, H., Bonato, P., Chan, L., & Rodgers, M. (2012). A review of wearable sensors and systems with application in rehabilitation. Journal of neuroengineering and rehabilitation, 9(1), 21.
[6] Dietterich, T. G. (2000). An overview of machine learning. Computer Science, 1, 4-15.
[7] Pearl, J. (2009). Causality. Cambridge university press.
[8] Christakis, N. A., & Fowler, J. H. (2007). The spread of obesity in a large social network over 32 years. New England Journal of Medicine, 357(4), 370-379.
[9] Nadkarni, P. M., Ohno-Machado, L., & Chapman, W. W. (2011). Natural language processing and electronic health records. Journal of the American Medical Informatics Association, 18(5), 544-551.
[10] US Department of Health and Human Services. (n.d.). HIPAA. Retrieved from https://www.hhs.gov/hipaa/index.html
[11] O’Neil, C. (2016). Weapons of math destruction: How big data increases inequality and threatens democracy. Crown.
[12] National Institute on Drug Abuse. (2020). Principles of drug addiction treatment: A research-based guide (Third Edition). NIH.
[13] Wang, R., Chen, F., Chen, Z., Li, R., & Lv, J. (2019). Machine learning for relapse prediction in substance use disorders: A systematic review. Addictive Behaviors, 99, 106084.
[14] Friedmann, P. D., Jiang, H., Gordon, A. J., Barry, D. T., & Ziedonis, D. M. (2006). Effectiveness of collaborative care for addiction in primary care settings. Journal of the American Medical Association, 296(10), 1239-1247.
[15] Adler-Milstein, J., & Bates, D. W. (2010). Creative destruction and the centrality of the electronic health record. Health Affairs, 29(7), 1147-1156.
[16] Gerke, S., Minssen, T., & Cohen, G. (2020). The need for a data science code of ethics. Nature Machine Intelligence, 2(1), 1-3.
[17] Eccles, M. P., & Mittman, B. S. (2006). Welcome to implementation science. Implementation Science, 1(1), 1.
Be the first to comment