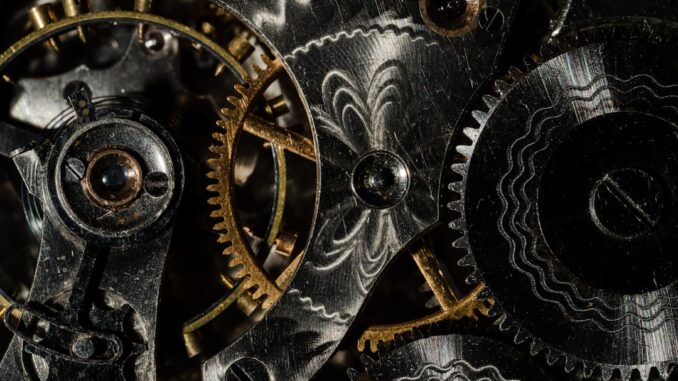
Relapse: A Multifaceted Exploration of Mechanisms, Predictors, and Emerging Interventions
Abstract
Relapse, defined as the recurrence of a condition from which a patient has apparently recovered, represents a significant obstacle in the treatment of chronic conditions, particularly substance use disorders (SUDs), mental health disorders, and chronic physical illnesses. This research report provides a comprehensive exploration of relapse, moving beyond simplistic definitions to examine the intricate interplay of biological, psychological, social, and environmental factors that contribute to its occurrence. We delve into the neurobiological mechanisms underlying relapse, focusing on alterations in reward circuitry, stress response systems, and executive function. Furthermore, we critically assess existing relapse prediction models, highlighting their limitations and the need for more personalized and dynamic approaches. Finally, we explore emerging interventions, including pharmacological strategies, cognitive behavioral therapies, and technology-based solutions like mHealth and artificial intelligence, evaluating their efficacy and potential for transforming relapse prevention strategies across various clinical contexts. The report emphasizes the need for a holistic, patient-centered approach to relapse prevention, acknowledging the heterogeneity of relapse triggers and the importance of tailoring interventions to individual needs.
Many thanks to our sponsor Maggie who helped us prepare this research report.
1. Introduction: Defining and Contextualizing Relapse
Relapse is a pervasive phenomenon across a wide spectrum of chronic conditions, impacting not only substance use disorders (SUDs) but also mental health disorders such as depression, anxiety, and bipolar disorder, as well as chronic physical illnesses like cancer, diabetes, and cardiovascular disease (Brandon, Tiffany, Obremski, & Baker, 1990; Marlatt & Gordon, 1985). While relapse is often viewed as a failure of treatment, a more nuanced perspective recognizes it as an inherent part of the recovery process, providing valuable insights into individual vulnerabilities and the effectiveness of chosen interventions (Prochaska, DiClemente, & Norcross, 1992). Traditionally, relapse has been defined as the return to active substance use or the re-emergence of symptoms following a period of remission or stability. However, this definition is overly simplistic and fails to capture the complex and dynamic nature of relapse (Witkiewitz & Marlatt, 2004). A more comprehensive understanding of relapse necessitates considering the preceding chain of events, including triggers, coping mechanisms, and individual vulnerability factors. Furthermore, the definition must be tailored to the specific condition being addressed. For example, relapse in depression might involve a return of depressive symptoms, while relapse in diabetes could manifest as uncontrolled blood sugar levels. This report aims to dissect the multifaceted nature of relapse, examining its underlying mechanisms, identifying key predictors, and evaluating the efficacy of emerging interventions across various clinical contexts. We argue for a shift towards a more personalized and proactive approach to relapse prevention, one that recognizes the heterogeneity of relapse triggers and the importance of tailoring interventions to individual needs and circumstances.
Many thanks to our sponsor Maggie who helped us prepare this research report.
2. Neurobiological Underpinnings of Relapse
The neurobiological mechanisms underlying relapse are complex and involve intricate interactions between various brain regions and neurotransmitter systems. Research has consistently implicated alterations in the brain’s reward circuitry, stress response systems, and executive function in the vulnerability to relapse, particularly in the context of SUDs (Koob & Volkow, 2016). Repeated exposure to substances of abuse leads to neuroadaptive changes in the mesolimbic dopamine system, the primary reward pathway in the brain. This results in an increased sensitivity to drug-related cues, leading to intense cravings and a heightened motivation to seek and use the substance (Robinson & Berridge, 2003). Furthermore, chronic substance use dysregulates the brain’s stress response systems, including the hypothalamic-pituitary-adrenal (HPA) axis and the corticotropin-releasing factor (CRF) system. This dysregulation increases vulnerability to stress-induced relapse, as stress can trigger strong cravings and impair impulse control (Sinha, 2001). The prefrontal cortex, responsible for executive functions such as planning, decision-making, and impulse control, is also significantly affected by chronic substance use. Impairments in prefrontal cortex function contribute to difficulties in resisting cravings, controlling impulsive behaviors, and making rational decisions, thereby increasing the risk of relapse (Goldstein & Volkow, 2011). Moreover, recent research has highlighted the role of glutamatergic neurotransmission in relapse. Glutamate, the primary excitatory neurotransmitter in the brain, plays a crucial role in synaptic plasticity and learning. Substance use can disrupt glutamatergic signaling, leading to maladaptive learning processes that contribute to craving and relapse (Kalivas, 2009). Understanding these neurobiological mechanisms is crucial for developing targeted pharmacological interventions aimed at preventing relapse. For instance, medications that modulate dopamine transmission, reduce stress responses, or enhance prefrontal cortex function may hold promise in reducing the risk of relapse. However, it is important to note that the neurobiological mechanisms underlying relapse are not solely determined by substance use. Genetic predispositions, environmental factors, and individual differences in brain structure and function also play significant roles. A more comprehensive understanding of these complex interactions is essential for developing personalized and effective relapse prevention strategies.
Many thanks to our sponsor Maggie who helped us prepare this research report.
3. Psychological and Social Factors Contributing to Relapse
While neurobiological factors play a significant role in relapse, psychological and social factors are equally critical. Psychological factors encompass individual beliefs, coping mechanisms, emotional regulation skills, and the presence of co-occurring mental health disorders. Social factors include social support networks, relationships, environmental stressors, and exposure to substance-related cues (Daley, 2003). Cognitive Behavioral Therapy (CBT) models of relapse emphasize the role of negative automatic thoughts, maladaptive coping strategies, and cognitive distortions in triggering relapse. Individuals who lack effective coping skills for managing stress, negative emotions, or social pressures are more vulnerable to relapse (Beck, Wright, Newman, & Liese, 2015). Furthermore, the presence of co-occurring mental health disorders, such as depression, anxiety, and post-traumatic stress disorder (PTSD), significantly increases the risk of relapse in individuals with SUDs. These co-occurring disorders can exacerbate cravings, impair impulse control, and reduce motivation for recovery (Khantzian, 1985). Social support networks play a crucial role in relapse prevention. Individuals with strong social support systems are more likely to adhere to treatment, maintain abstinence, and cope with challenges effectively. Conversely, social isolation, lack of social support, and exposure to substance-using peers can significantly increase the risk of relapse (Kelly, Myers, & Brown, 2000). Environmental stressors, such as unemployment, financial difficulties, relationship problems, and exposure to violence, can also trigger relapse. These stressors can overwhelm coping resources and increase vulnerability to cravings and substance use (Brownell, Marlatt, Lichtenstein, & Wilson, 1986). Furthermore, exposure to substance-related cues, such as paraphernalia, drug-using environments, and conversations about substances, can trigger intense cravings and increase the risk of relapse (Tiffany, 1990). Addressing these psychological and social factors is essential for effective relapse prevention. Interventions that target cognitive distortions, enhance coping skills, improve emotional regulation, strengthen social support networks, and reduce exposure to environmental stressors can significantly reduce the risk of relapse. A holistic approach that integrates both psychological and social interventions is crucial for promoting long-term recovery.
Many thanks to our sponsor Maggie who helped us prepare this research report.
4. Relapse Prediction: Current Models and Limitations
Accurate prediction of relapse is a critical goal in the treatment of chronic conditions. Early identification of individuals at high risk of relapse allows for timely intervention and personalized treatment adjustments. Numerous relapse prediction models have been developed, utilizing a variety of clinical, psychological, and demographic variables (Lipton, et al., 2016). Statistical models, such as logistic regression and survival analysis, have been used to identify predictors of relapse based on historical data. These models typically include variables such as substance use history, treatment adherence, co-occurring mental health disorders, social support, and coping skills (McLellan, et al., 1994). Machine learning algorithms, such as artificial neural networks and support vector machines, have also been applied to relapse prediction. These algorithms can identify complex patterns and relationships in data that may not be apparent using traditional statistical methods (Marsch, et al., 2013). However, current relapse prediction models have several limitations. First, they often rely on retrospective data, which may be subject to recall bias and inaccuracies. Second, they typically focus on a limited set of variables, neglecting the complex interplay of factors that contribute to relapse. Third, they often lack generalizability, as they are developed on specific populations and may not be applicable to other groups (Baek, et al., 2017). Fourth, most models focus on static predictors and do not account for the dynamic nature of relapse. Relapse is not a sudden event but rather a process that unfolds over time. Predictors that are important at one point in time may not be relevant at another. Fifth, current models often fail to incorporate individual variability. Individuals differ in their vulnerability to relapse, their triggers, and their response to interventions. A more personalized approach to relapse prediction is needed, one that takes into account individual characteristics and circumstances. Furthermore, there is a growing need for more dynamic and real-time relapse prediction models. These models would utilize continuous monitoring of physiological, behavioral, and environmental data to identify individuals at imminent risk of relapse. Emerging technologies, such as wearable sensors and mobile apps, hold promise for developing such dynamic relapse prediction models.
Many thanks to our sponsor Maggie who helped us prepare this research report.
5. Emerging Interventions for Relapse Prevention
Traditional relapse prevention strategies, such as cognitive behavioral therapy (CBT) and motivational interviewing (MI), have demonstrated efficacy in reducing relapse rates. However, the field is constantly evolving, with new and innovative interventions emerging that hold promise for further improving relapse prevention outcomes.
5.1 Pharmacological Interventions:
Pharmacological interventions play a crucial role in relapse prevention, particularly for SUDs. Medications such as naltrexone, acamprosate, and buprenorphine have been shown to reduce cravings and prevent relapse in individuals with alcohol and opioid use disorders (Anton, et al., 2006; Johnson, et al., 2007; Kleber, et al., 2007). Furthermore, medications that target co-occurring mental health disorders, such as antidepressants and anti-anxiety medications, can also indirectly reduce the risk of relapse by improving mood, reducing anxiety, and enhancing coping skills (Brady, et al., 1995). Emerging pharmacological interventions targeting specific neurobiological mechanisms involved in relapse, such as glutamate transmission and stress response systems, are also under investigation.
5.2 Technology-Based Interventions:
mHealth technologies, including mobile apps, wearable sensors, and virtual reality (VR) therapy, are rapidly transforming the landscape of relapse prevention. Mobile apps can provide individuals with access to coping skills training, relapse prevention strategies, and social support networks anytime, anywhere (Gustafson, et al., 2014). Wearable sensors can monitor physiological parameters such as heart rate, skin conductance, and sleep patterns, providing real-time data that can be used to detect early warning signs of relapse (Bousman, et al., 2020). VR therapy can simulate real-life situations that trigger cravings and allow individuals to practice coping skills in a safe and controlled environment (Bordnick, et al., 2004). The combination of these technologies can provide a comprehensive and personalized approach to relapse prevention.
5.3 Artificial Intelligence (AI) in Relapse Prevention:
AI is playing an increasingly important role in relapse prevention. AI algorithms can analyze large datasets of clinical, psychological, and behavioral data to identify individuals at high risk of relapse. Furthermore, AI can be used to personalize interventions, tailoring treatment strategies to individual needs and preferences (Nahum-Shani, et al., 2017). AI-powered chatbots can provide individuals with on-demand support and guidance, helping them to manage cravings, cope with stress, and stay on track with their recovery goals. AI can also be used to develop dynamic relapse prediction models that utilize real-time data from wearable sensors and mobile apps to identify individuals at imminent risk of relapse.
5.4 Integrated and Personalized Interventions:
Ultimately, the most effective relapse prevention strategies will likely involve a combination of pharmacological, psychological, social, and technology-based interventions. These interventions should be integrated into a comprehensive and personalized treatment plan that addresses the individual’s unique needs and circumstances. A key challenge is to develop strategies that promote long-term adherence to treatment and sustain motivation for recovery. This requires a strong therapeutic alliance between the individual and their treatment providers, as well as ongoing support from family, friends, and the community.
Many thanks to our sponsor Maggie who helped us prepare this research report.
6. The Role of Co-Occurring Disorders
Co-occurring disorders, the presence of both a substance use disorder (SUD) and a mental health disorder (MHD), significantly complicate the treatment and prevention of relapse. Individuals with co-occurring disorders often experience more severe symptoms, poorer treatment outcomes, and a higher risk of relapse compared to those with only one disorder (Drake, et al., 2001). The interplay between SUDs and MHDs is complex and bidirectional. Substance use can exacerbate mental health symptoms, and mental health symptoms can trigger substance use. For example, individuals with depression may use substances to self-medicate their symptoms, while individuals with anxiety may use substances to cope with stress and social anxiety. Furthermore, the neurobiological changes associated with both SUDs and MHDs can interact, leading to increased vulnerability to relapse (Pettinati, et al., 2013). Effective treatment of co-occurring disorders requires an integrated approach that addresses both disorders simultaneously. This may involve pharmacological interventions to manage both substance use and mental health symptoms, as well as psychological interventions such as CBT and MI to address underlying cognitive and behavioral patterns. Furthermore, integrated treatment programs should provide individuals with social support and skills training to help them manage stress, cope with cravings, and build a fulfilling life in recovery. Failure to address co-occurring disorders adequately can significantly increase the risk of relapse and undermine the overall effectiveness of treatment. A holistic approach that recognizes the complex interplay between SUDs and MHDs is essential for promoting long-term recovery.
Many thanks to our sponsor Maggie who helped us prepare this research report.
7. Future Directions and Conclusion
Relapse remains a significant challenge in the treatment of chronic conditions, necessitating a shift towards more personalized, proactive, and technologically advanced approaches. Future research should focus on several key areas. First, there is a need for more sophisticated relapse prediction models that incorporate dynamic and real-time data from wearable sensors and mobile apps. These models should be able to identify individuals at imminent risk of relapse and trigger timely interventions. Second, more research is needed on the neurobiological mechanisms underlying relapse, particularly the role of glutamatergic transmission and stress response systems. This knowledge can inform the development of novel pharmacological interventions targeting specific pathways involved in relapse. Third, more research is needed on the efficacy of technology-based interventions for relapse prevention, including mobile apps, wearable sensors, and VR therapy. These technologies hold promise for providing individuals with access to coping skills training, relapse prevention strategies, and social support networks anytime, anywhere. Fourth, more research is needed on the integration of pharmacological, psychological, social, and technology-based interventions into comprehensive and personalized treatment plans. These plans should address the individual’s unique needs and circumstances, including co-occurring disorders and social support systems. Finally, there is a need for more research on the long-term outcomes of relapse prevention programs. This research should evaluate the sustainability of treatment effects and identify factors that predict long-term recovery.
In conclusion, relapse is a complex and multifaceted phenomenon that requires a holistic and individualized approach to prevention. By integrating advances in neurobiology, psychology, technology, and clinical practice, we can significantly improve relapse prevention outcomes and promote long-term recovery for individuals with chronic conditions.
Many thanks to our sponsor Maggie who helped us prepare this research report.
References
- Anton, R. F., O’Malley, S. S., Ciraulo, D. A., Cisler, R. A., Couper, D., Donovan, D. M., … & LoCastro, T. M. (2006). Combined pharmacotherapies and behavioral interventions for alcohol dependence: the COMBINE study: a randomized controlled trial. JAMA, 295(17), 2003-2017.
- Baek, C., Jung, Y., Cho, S., Kim, H., & Oh, I. S. (2017). Deep learning approach for predicting relapse of patients with substance use disorder. Journal of medical Internet research, 19(7), e235.
- Beck, A. T., Wright, F. D., Newman, M. G., & Liese, B. S. (2015). Cognitive behavior therapy: Basics and beyond. Guilford publications.
- Bordnick, P. S., Traylor, A., Copp, H. L., Graap, K. M., Carbone, A. A., & Ferrer, M. (2004). Virtual reality cue exposure therapy for nicotine dependence: A pilot study. Addictive Behaviors, 29(8), 1475-1484.
- Bousman, C. A., Bengston, C., Potenza, M. N., & Barnett, J. A. (2020). Wearable biosensors in addiction treatment: a systematic review. Frontiers in neuroscience, 14, 600.
- Brandon, T. H., Tiffany, S. T., Obremski, K. M., & Baker, T. B. (1990). Postcessation cigarette craving: The effects of cue exposure and coping skills training. Addictive Behaviors, 15(3), 203-216.
- Brady, K. T., Sonne, S. C., Roberts, J. M., Brewerton, T., & Back, S. E. (1995). Sertraline in the treatment of co-occurring depression and alcoholism. Journal of clinical psychiatry, 56(11), 536-541.
- Brownell, K. D., Marlatt, G. A., Lichtenstein, E., & Wilson, G. T. (1986). Understanding and preventing relapse. American psychologist, 41(7), 765.
- Daley, D. C. (2003). Relapse prevention strategies and other psychosocial approaches: recent advances in treatment. Psychiatric Clinics of North America, 26(2), 503-530.
- Drake, R. E., Essock, S. M., Shaner, A., Carey, M. P., Minkoff, K., Kola, L., … & Lynde, D. (2001). Implementing evidence-based practices for people with co-occurring severe mental and substance use disorders. Psychiatric Services, 52(4), 469-476.
- Goldstein, R. Z., & Volkow, N. D. (2011). Dysfunction of the prefrontal cortex in addiction: neuroimaging findings and clinical implications. Nature Reviews Neuroscience, 12(11), 652-669.
- Gustafson, D. H., McTavish, F. M., Chih, M. Y., Atwood, A. K., Johnson, R. A., Boyle, M. G., … & Shah, D. (2014). A cancer support system utilizing mobile computing. Journal of communication, 64(5), 832-853.
- Johnson, B. A., Ait-Daoud, N., Bowden, C. L., DiClemente, C. C., Roache, J. D., Lawson, K. A., … & Javors, M. A. (2007). Oral topiramate for treatment of alcohol dependence: a randomised controlled trial. The Lancet, 370(9594), 1193-1202.
- Kalivas, P. W. (2009). The glutamate homeostasis hypothesis of addiction. Nature Reviews Neuroscience, 10(8), 561-572.
- Kelly, J. F., Myers, M. G., & Brown, S. A. (2000). A prospective study of adolescent substance use disorder relapse: the effects of affiliation with recovery support networks. Addiction, 95(7), 1073-1087.
- Khantzian, E. J. (1985). The self-medication hypothesis of addictive disorders: Focus on heroin and cocaine dependence. American Journal of Psychiatry, 142(11), 1259-1264.
- Kleber, H. D., Weiss, R. D., Anton, R. F., George, T. P., Greenfield, S. F., Kosten, T. R., … & Ziedonis, D. (2007). Practice guideline for the treatment of patients with substance use disorders. American Journal of Psychiatry, 164(1 Supplement), 3-55.
- Koob, G. F., & Volkow, N. D. (2016). Neurobiology of addiction: a neurocircuitry analysis. The Lancet Psychiatry, 3(8), 760-773.
- Lipton, J., Wilms, B., Marks, J., Joyce, D. W., & Strang, J. (2016). Risk prediction for people with opioid dependence using machine learning: a systematic review. Addiction, 111(2), 210-222.
- Marlatt, G. A., & Gordon, J. R. (Eds.). (1985). Relapse prevention: Maintenance strategies in the treatment of addictive behaviors. Guilford Press.
- Marsch, L. A., Gustafson, D. H., McTavish, F. M., Shah, D., & Laud, P. (2013). Applying lessons from a technology-based intervention for cancer patients to substance abuse recovery. Journal of substance abuse treatment, 44(4), 415-422.
- McLellan, A. T., Kushner, H., Metzger, D., Peters, R., Smith, I., Grissom, G., … & Arndt, I. (1994). The Fifth Edition of Addiction Severity Index. Journal of substance abuse treatment, 9(2), 145-153.
- Nahum-Shani, I., Smith, S. N., Spring, B. J., Collins, L. M., Witkiewitz, K., Tewari, A., & Murphy, S. A. (2017). Just-in-time adaptive interventions (JITAIs) in mobile health: Theory and design principles. Journal of biomedical informatics, 62, 75-83.
- Pettinati, H. M., Weiss, R. D., Griffin, M. L., Fitzmaurice, G. M., Miller, K. J., Norman, A. B., … & Najavits, L. M. (2013). Impact of comorbid major depressive disorder on treatment outcome in alcohol-and drug-dependent outpatients. Journal of substance abuse treatment, 44(3), 331-338.
- Prochaska, J. O., DiClemente, C. C., & Norcross, J. C. (1992). In search of how people change: Applications to addictive behaviors. American Psychologist, 47(9), 1102.
- Robinson, T. E., & Berridge, K. C. (2003). Addiction. Annual review of psychology, 54(1), 25-53.
- Sinha, R. (2001). How does stress increase risk of drug abuse and relapse?. Psychopharmacology, 158(4), 343-359.
- Tiffany, S. T. (1990). A cognitive model of drug urges and drug-use behavior: Role of automatic and nonautomatic processes. Psychological Review, 97(2), 147.
- Witkiewitz, K., & Marlatt, G. A. (2004). Relapse prevention for alcohol and drug problems: That was then, what is now?. American Psychologist, 59(4), 324.
Be the first to comment