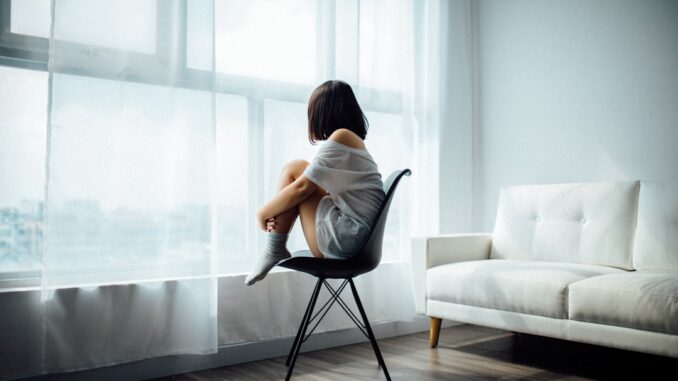
Abstract
Depression, a pervasive and debilitating mental disorder, presents a significant global health challenge. This research report provides a comprehensive overview of depression, exploring its diagnosis, etiological underpinnings, social and economic impacts, treatment modalities, and emerging prevention strategies. A critical focus is placed on the advancements in artificial intelligence (AI) for depression prediction, specifically addressing the existing racial and cultural disparities in the accuracy and applicability of these models. The report delves into the methodological challenges associated with cross-cultural assessment and highlights the imperative need for culturally sensitive diagnostic tools and interventions to mitigate health inequities. Furthermore, it examines the ethical considerations surrounding the use of AI in mental health, emphasizing the importance of transparency, fairness, and accountability to ensure equitable and effective mental healthcare delivery.
Many thanks to our sponsor Maggie who helped us prepare this research report.
1. Introduction
Major Depressive Disorder (MDD), commonly known as depression, affects millions worldwide, contributing significantly to disability, morbidity, and mortality. It is characterized by persistent feelings of sadness, loss of interest or pleasure, fatigue, sleep disturbances, and difficulties in concentration, significantly impacting an individual’s ability to function in daily life (American Psychiatric Association, 2013). The World Health Organization (WHO) recognizes depression as a leading cause of disability, emphasizing the urgent need for effective diagnosis, treatment, and prevention strategies (WHO, 2023).
Understanding depression requires a multifaceted approach, considering its complex etiology, diverse clinical presentations, and the influence of social, cultural, and environmental factors. Traditional diagnostic methods, relying primarily on clinical interviews and standardized questionnaires, can be subjective and prone to biases, particularly when applied across diverse populations. Recent advancements in AI and machine learning offer promising avenues for improving the accuracy and efficiency of depression diagnosis and prediction, potentially revolutionizing mental healthcare delivery (Inkster et al., 2016). However, these technologies also raise concerns about algorithmic bias, data privacy, and the potential for exacerbating existing health disparities. Therefore, a critical and nuanced examination of the current state of depression research and the ethical implications of AI-driven interventions is essential. This report aims to provide such an examination, offering a comprehensive overview of the latest research, challenges, and future directions in the field.
Many thanks to our sponsor Maggie who helped us prepare this research report.
2. Diagnostic Criteria and Methods
The Diagnostic and Statistical Manual of Mental Disorders (DSM-5) outlines the criteria for diagnosing MDD (American Psychiatric Association, 2013). These criteria involve the presence of five or more symptoms during a two-week period, with at least one symptom being either depressed mood or loss of interest or pleasure. Other symptoms include significant weight loss or gain, insomnia or hypersomnia, psychomotor agitation or retardation, fatigue or loss of energy, feelings of worthlessness or excessive guilt, diminished ability to think or concentrate, and recurrent thoughts of death or suicide.
Diagnosis typically involves a clinical interview, during which a mental health professional assesses the individual’s symptoms, medical history, and social context. Standardized questionnaires, such as the Beck Depression Inventory (BDI), the Hamilton Rating Scale for Depression (HRSD), and the Patient Health Questionnaire-9 (PHQ-9), are commonly used to quantify symptom severity and track treatment progress (Beck et al., 1961; Hamilton, 1960; Kroenke et al., 2001). Neuroimaging techniques, such as functional magnetic resonance imaging (fMRI) and electroencephalography (EEG), are increasingly being used to identify neural correlates of depression and to differentiate between subtypes of the disorder (Gotlib & Hamilton, 2008). However, these techniques are primarily used in research settings and are not yet routinely employed in clinical practice. The use of biomarkers, such as cortisol levels and inflammatory markers, has also been investigated as potential diagnostic tools, but their clinical utility remains limited (Halaris, 2003).
2.1 Challenges in Cross-Cultural Diagnosis
Diagnosing depression across different cultural groups presents significant challenges. Cultural variations in symptom expression, help-seeking behavior, and access to mental healthcare can lead to underdiagnosis or misdiagnosis (Lewis-Fernández et al., 2002). For example, individuals from some cultures may somatize their emotional distress, presenting primarily with physical symptoms rather than psychological ones. Stigma surrounding mental illness can also prevent individuals from seeking help or disclosing their symptoms accurately (Corrigan, 2004). Moreover, the validity of standardized diagnostic tools may vary across cultures, as items may not be culturally relevant or understood in the same way. For instance, questions about guilt or worthlessness may have different meanings in collectivist cultures compared to individualistic cultures. Therefore, it is crucial to use culturally adapted assessment tools and to consider the individual’s cultural background when interpreting symptoms.
2.2 The Promise and Perils of AI in Diagnosis
AI and machine learning hold immense potential for improving the accuracy and efficiency of depression diagnosis. Machine learning algorithms can analyze large datasets of clinical data, including patient records, neuroimaging data, and social media activity, to identify patterns and predictors of depression (Inkster et al., 2016). Natural language processing (NLP) can be used to analyze text and speech data to detect subtle changes in language patterns that may indicate depression (Low et al., 2020). AI-powered chatbots and virtual assistants can provide accessible and affordable mental health support, particularly in underserved communities (Fitzpatrick et al., 2017).
However, the use of AI in depression diagnosis also raises ethical concerns. Algorithmic bias, resulting from biased training data, can lead to inaccurate or unfair predictions, particularly for underrepresented groups (Buolamwini & Gebru, 2018). For instance, if an AI model is trained primarily on data from white individuals, it may not accurately diagnose depression in individuals from other racial or ethnic groups. Data privacy and security are also major concerns, as sensitive mental health data must be protected from unauthorized access and misuse. Furthermore, the lack of transparency and explainability of some AI models can make it difficult to understand how they arrive at their predictions, raising questions about accountability and trust.
Therefore, the development and deployment of AI-driven diagnostic tools must be guided by ethical principles, including fairness, transparency, accountability, and respect for privacy. It is crucial to use diverse and representative training datasets, to validate AI models across different populations, and to ensure that AI systems are used to augment, rather than replace, human expertise.
Many thanks to our sponsor Maggie who helped us prepare this research report.
3. Etiology and Pathophysiology
The etiology of depression is complex and multifactorial, involving a combination of genetic, biological, psychological, and environmental factors (Kendler et al., 2006). While genetic factors contribute significantly to the risk of developing depression, they do not fully determine an individual’s vulnerability. Epigenetic mechanisms, which involve changes in gene expression without alterations in the DNA sequence, may also play a role in the development of depression (McGowan et al., 2009). Adverse childhood experiences, such as abuse, neglect, and trauma, can increase the risk of depression later in life, possibly through epigenetic modifications.
3.1 Neurobiological Mechanisms
Neurobiological research has identified several brain regions and neurotransmitter systems that are implicated in the pathophysiology of depression. The monoamine hypothesis, which posits that depression is caused by a deficiency of serotonin, norepinephrine, and dopamine, has been a dominant framework for understanding the neurochemical basis of depression (Schildkraut, 1965). However, this hypothesis is an oversimplification, as many individuals with depression do not respond to medications that increase monoamine levels. Other neurotransmitters, such as glutamate and GABA, have also been implicated in the pathophysiology of depression (Sanacora et al., 2008).
Neuroimaging studies have revealed structural and functional abnormalities in several brain regions in individuals with depression, including the prefrontal cortex, amygdala, hippocampus, and anterior cingulate cortex (Drevets et al., 2008). The prefrontal cortex is involved in executive functions, such as planning and decision-making, and its dysfunction may contribute to the cognitive impairments associated with depression. The amygdala is involved in processing emotions, and its hyperactivity may contribute to the negative emotions associated with depression. The hippocampus is involved in memory, and its atrophy may contribute to the memory problems associated with depression. The anterior cingulate cortex is involved in regulating emotions and behavior, and its dysfunction may contribute to the difficulty in regulating emotions associated with depression.
3.2 The Role of Stress and Inflammation
Chronic stress is a major risk factor for depression. Stressful life events can trigger the onset of depression in vulnerable individuals (Kendler et al., 2006). The hypothalamic-pituitary-adrenal (HPA) axis, which is responsible for regulating the stress response, is often dysregulated in individuals with depression. Chronic activation of the HPA axis can lead to elevated levels of cortisol, which can have detrimental effects on the brain and body.
Inflammation has also been implicated in the pathophysiology of depression. Studies have shown that individuals with depression have higher levels of inflammatory markers, such as C-reactive protein (CRP) and interleukin-6 (IL-6), in their blood (Dowlati et al., 2010). Inflammation may contribute to depression by disrupting neurotransmitter function, damaging brain cells, and impairing neuroplasticity. Targeting inflammation may be a promising avenue for developing new treatments for depression.
Many thanks to our sponsor Maggie who helped us prepare this research report.
4. Social and Economic Impacts
Depression has profound social and economic consequences, affecting individuals, families, and communities. Individuals with depression experience significant impairments in their ability to work, study, and maintain social relationships. Depression is a leading cause of absenteeism and presenteeism in the workplace, resulting in lost productivity and economic costs (Greenberg et al., 2003). Depression can also strain family relationships, leading to conflict, separation, and divorce. Children of parents with depression are at increased risk of developing mental health problems themselves.
The economic burden of depression is substantial. Depression is associated with increased healthcare costs, including costs for medication, therapy, and hospitalization (Greenberg et al., 2003). Lost productivity due to absenteeism and presenteeism also contributes significantly to the economic costs of depression. In addition, depression is associated with increased rates of suicide, which is a tragic and preventable cause of death. The economic costs of suicide are difficult to quantify, but they include lost earnings, healthcare costs, and the emotional toll on families and communities.
4.1 Addressing the Social Determinants of Mental Health
Addressing the social determinants of mental health is crucial for preventing and treating depression. Social determinants of mental health include factors such as poverty, unemployment, lack of access to education and healthcare, discrimination, and social isolation. These factors can increase the risk of depression and can make it more difficult to recover from the disorder. Addressing these social determinants requires a multi-pronged approach, involving policies and programs that promote economic opportunity, improve access to education and healthcare, reduce discrimination, and foster social inclusion. Community-based interventions, such as peer support groups and social support networks, can also play a vital role in promoting mental health and well-being.
Many thanks to our sponsor Maggie who helped us prepare this research report.
5. Treatment Modalities
Numerous effective treatments are available for depression, including psychotherapy, pharmacotherapy, and brain stimulation therapies (National Institute for Health and Care Excellence, 2021). The choice of treatment depends on the severity of the depression, the individual’s preferences, and the presence of comorbid conditions.
5.1 Psychotherapy
Psychotherapy, also known as talk therapy, involves working with a therapist to address the emotional, cognitive, and behavioral aspects of depression. Cognitive behavioral therapy (CBT) is a widely used and effective form of psychotherapy for depression (Beck, 1976). CBT focuses on identifying and changing negative thought patterns and behaviors that contribute to depression. Interpersonal therapy (IPT) is another effective form of psychotherapy for depression (Klerman et al., 1984). IPT focuses on improving interpersonal relationships and addressing social stressors that contribute to depression. Other forms of psychotherapy, such as psychodynamic therapy and mindfulness-based therapy, may also be helpful for some individuals with depression.
5.2 Pharmacotherapy
Antidepressant medications are commonly used to treat depression. Selective serotonin reuptake inhibitors (SSRIs) are a first-line treatment for depression due to their relatively mild side effects (Wong et al., 1995). SSRIs work by increasing the levels of serotonin in the brain. Other types of antidepressants include serotonin-norepinephrine reuptake inhibitors (SNRIs), tricyclic antidepressants (TCAs), and monoamine oxidase inhibitors (MAOIs). The choice of antidepressant depends on the individual’s symptoms, medical history, and potential side effects. Antidepressant medications can be effective in reducing the symptoms of depression, but they may not be effective for everyone. Some individuals may experience side effects from antidepressants, such as nausea, insomnia, and sexual dysfunction. It is important to work closely with a healthcare provider to find the right medication and dosage.
5.3 Brain Stimulation Therapies
Brain stimulation therapies, such as electroconvulsive therapy (ECT) and transcranial magnetic stimulation (TMS), are used to treat severe depression that has not responded to other treatments. ECT involves passing an electrical current through the brain to induce a seizure. ECT is a highly effective treatment for depression, but it can have side effects, such as memory loss. TMS involves using magnetic pulses to stimulate specific areas of the brain. TMS is a non-invasive treatment that is generally well-tolerated, but it may not be as effective as ECT.
5.4 Culturally Sensitive Interventions
Culturally sensitive interventions are essential for providing effective mental healthcare to diverse populations. These interventions take into account the individual’s cultural background, beliefs, and values. Culturally adapted therapies may involve modifying existing therapies to make them more culturally relevant or developing new therapies that are specifically tailored to the needs of a particular cultural group (Bernal et al., 1995). Culturally sensitive interventions also address the social determinants of mental health, such as poverty, discrimination, and lack of access to education and healthcare. Community-based interventions, such as peer support groups and cultural centers, can also play a vital role in promoting mental health and well-being in diverse communities.
Many thanks to our sponsor Maggie who helped us prepare this research report.
6. Prevention Strategies
Preventing depression is a public health priority. Prevention strategies can target individuals at high risk of developing depression, such as those with a family history of depression or those who have experienced adverse childhood experiences. Prevention strategies can also target the general population, promoting mental health and well-being through education, awareness campaigns, and social support programs.
6.1 Early Intervention Programs
Early intervention programs can help prevent depression in children and adolescents at high risk of developing the disorder. These programs may involve teaching coping skills, promoting positive social interactions, and addressing family stressors. School-based programs can also play a vital role in preventing depression by promoting mental health awareness and providing access to mental health services.
6.2 Lifestyle Interventions
Lifestyle interventions, such as exercise, healthy diet, and stress management techniques, can help prevent depression in adults. Regular exercise has been shown to have antidepressant effects (Blumenthal et al., 1999). A healthy diet, rich in fruits, vegetables, and whole grains, can also promote mental health. Stress management techniques, such as yoga, meditation, and mindfulness, can help reduce stress and improve mood.
6.3 Social Support Networks
Strong social support networks can protect against depression. Maintaining close relationships with family and friends can provide emotional support and reduce feelings of isolation. Participating in community activities and volunteering can also promote social connectedness and well-being. Addressing social isolation and promoting social inclusion are important strategies for preventing depression.
Many thanks to our sponsor Maggie who helped us prepare this research report.
7. The Future of Depression Research and Treatment
The future of depression research and treatment holds great promise. Advances in neuroscience, genetics, and technology are paving the way for new and more effective treatments. Personalized medicine, which involves tailoring treatment to the individual’s specific needs and characteristics, is a promising approach for improving treatment outcomes.
7.1 Advancements in Neuroimaging and Biomarkers
Neuroimaging techniques, such as fMRI and EEG, are becoming increasingly sophisticated and are providing new insights into the neural mechanisms of depression. Biomarkers, such as genetic markers, inflammatory markers, and neuroendocrine markers, are also being investigated as potential diagnostic and prognostic tools. Combining neuroimaging and biomarker data may help to identify subtypes of depression and to predict treatment response.
7.2 The Role of Technology in Mental Healthcare
Technology is transforming mental healthcare, making it more accessible, affordable, and personalized. Telepsychiatry, which involves providing mental health services remotely using video conferencing or other technologies, can improve access to care for individuals in rural or underserved areas. Mobile health (mHealth) apps can provide self-monitoring tools, educational resources, and support for individuals with depression. Virtual reality (VR) therapy can be used to treat anxiety and phobias, which are often comorbid with depression. AI-powered chatbots and virtual assistants can provide accessible and affordable mental health support, particularly for individuals who are reluctant to seek traditional mental healthcare.
However, it is important to address the ethical and practical challenges associated with the use of technology in mental healthcare. Data privacy and security, algorithmic bias, and the digital divide are all important concerns that must be addressed to ensure that technology is used to promote equitable and effective mental healthcare.
7.3 Addressing Racial and Cultural Disparities in AI-Driven Predictive Models
The development and implementation of AI-driven predictive models for depression must prioritize the reduction of racial and cultural disparities. This requires several key steps:
- Diverse and Representative Datasets: AI models should be trained on datasets that accurately reflect the diversity of the population. This includes ensuring representation of different racial, ethnic, and cultural groups, as well as considering other demographic factors such as socioeconomic status and geographic location.
- Culturally Sensitive Feature Engineering: The features used to train AI models should be carefully selected and validated to ensure that they are culturally relevant and do not perpetuate existing biases. This may involve consulting with cultural experts and community members to identify culturally appropriate indicators of depression.
- Algorithmic Fairness: AI models should be evaluated for fairness across different racial and cultural groups. This involves using metrics that assess whether the model is making accurate and equitable predictions for all groups. Techniques such as adversarial training and fairness-aware machine learning can be used to mitigate algorithmic bias.
- Transparency and Explainability: AI models should be transparent and explainable, so that clinicians and patients can understand how they arrive at their predictions. This can help to build trust in the technology and to identify potential biases or errors.
- Community Engagement: The development and implementation of AI-driven predictive models should involve active engagement with community members, particularly those from underrepresented groups. This can help to ensure that the technology is culturally appropriate, ethically sound, and aligned with the needs of the community.
Many thanks to our sponsor Maggie who helped us prepare this research report.
8. Conclusion
Depression is a complex and debilitating mental disorder that affects millions worldwide. Understanding its multifaceted etiology, improving diagnostic accuracy, and developing effective treatments are crucial for reducing its impact on individuals, families, and communities. Advances in AI and technology offer promising avenues for improving mental healthcare delivery, but it is essential to address the ethical and practical challenges associated with their use. Culturally sensitive assessment tools and interventions are necessary to mitigate health inequities and to provide effective mental healthcare to diverse populations. By prioritizing ethical principles, promoting community engagement, and addressing the social determinants of mental health, we can create a future where depression is effectively prevented, diagnosed, and treated, and where all individuals have the opportunity to live fulfilling and meaningful lives. The path forward demands interdisciplinary collaboration, continuous evaluation, and a commitment to equitable and ethical innovation in mental health care.
Many thanks to our sponsor Maggie who helped us prepare this research report.
References
- American Psychiatric Association. (2013). Diagnostic and statistical manual of mental disorders (5th ed.).
- Beck, A. T. (1976). Cognitive therapy and the emotional disorders. International Universities Press.
- Beck, A. T., Ward, C. H., Mendelson, M., Mock, J., & Erbaugh, J. (1961). An inventory for measuring depression. Archives of General Psychiatry, 4(6), 561-571.
- Bernal, G., Bonilla, J., & Bellido, D. (1995). Ecological validity and cultural sensitivity for outcome research: Issues for the development and evaluation of psychosocial treatments with Hispanics. Journal of Abnormal Child Psychology, 23(1), 67-82.
- Blumenthal, J. A., Babyak, M. A., Moore, K. A., Craighead, W. E., Herman, S., Khatri, P., Waugh, R., Napolitano, M. A., Forman, L. M., Appelbaum, M., Doraiswamy, P. M., & Krishnan, K. R. (1999). Effects of exercise training on older patients with major depression. Archives of Internal Medicine, 159(19), 2349-2356.
- Buolamwini, J., & Gebru, T. (2018). Gender shades: Intersectional accuracy disparities in commercial gender classification. Proceedings of Machine Learning Research, 81, 1-15.
- Corrigan, P. W. (2004). How stigma interferes with mental health care. American Psychologist, 59(7), 614.
- Dowlati, Y., Herrmann, N., Swardfager, W., Thomson, K., Strawbridge, R., Mazereeuw, G., … & Lanctôt, K. L. (2010). A meta-analysis of cytokines in major depression. Biological Psychiatry, 67(5), 446-457.
- Drevets, W. C., Price, J. L., & Furey, M. L. (2008). Brain structural and functional abnormalities in mood disorders: Implications for neurocircuitry models of depression. Brain Structure and Function, 213(1-2), 93-118.
- Fitzpatrick, K. K., Darcy, A., & Vierhile, M. (2017). Delivering cognitive behavior therapy to young adults with symptoms of depression and anxiety using a fully automated conversational agent (Woebot): A randomized controlled trial. JMIR Mental Health, 4(2), e19.
- Gotlib, I. H., & Hamilton, J. P. (2008). Neuroimaging and depression: Current status and future directions. Current Directions in Psychological Science, 17(2), 119-123.
- Greenberg, P. E., Kessler, R. C., Birnbaum, H. G., Leong, S. A., Lowe, S. W., Berglund, P. A., & Corey-Lisle, P. K. (2003). The economic burden of depression in the United States: How did it change between 1990 and 2000?. Journal of Clinical Psychiatry, 64(12), 1465-1475.
- Halaris, A. (2003). Inflammation and depression: a dysregulation of the brain’s immune system. Psychiatric Clinics of North America, 26(3), 521-534.
- Hamilton, M. (1960). A rating scale for depression. Journal of Neurology, Neurosurgery & Psychiatry, 23(1), 56-62.
- Inkster, B., James, C. J., & Radua, J. (2016). Machine learning and mental health: where are we and where should we go?. Brain Informatics, 3(2), 125-143.
- Kendler, K. S., Kuhn, J. W., & Prescott, C. A. (2006). Childhood adversity, stressful life events, genetic liability, and risk for first-onset major depression. Archives of General Psychiatry, 63(12), 1322-1329.
- Klerman, G. L., Weissman, M. M., Rounsaville, B. J., & Chevron, E. S. (1984). Interpersonal psychotherapy of depression. Basic Books.
- Kroenke, K., Spitzer, R. L., & Williams, J. B. (2001). The PHQ-9: validity of a brief depression severity measure. Journal of General Internal Medicine, 16(9), 606-613.
- Lewis-Fernández, R., Kleinman, A., & Griffith, E. E. (2002). Cultural formulation. Psychiatric Clinics of North America, 25(3), 491-506.
- Low, D. M., Bentley, S. A., Ghosh, S. S., & Dunn, L. B. (2020). Natural language processing models for detecting depression: A systematic review. Journal of Affective Disorders, 268, 418-435.
- McGowan, P. O., Sasaki, A., D’Alessio, A. C., Dymov, S., Labonté, B., Meaney, M. J., & Szyf, M. (2009). Epigenetic regulation of the glucocorticoid receptor in human brain associates with childhood abuse. Nature Neuroscience, 12(3), 342-348.
- National Institute for Health and Care Excellence. (2021). Depression in adults: treatment and management. NICE guideline [NG222].
- Sanacora, G., Zarate Jr, C. A., Krystal, J. H., & Manji, H. K. (2008). Targeting the glutamatergic system to develop novel, improved therapeutics for mood disorders. Nature Reviews Drug Discovery, 7(5), 426-437.
- Schildkraut, J. J. (1965). The catecholamine hypothesis of affective disorders: a review of supporting evidence. American Journal of Psychiatry, 122(5), 509-522.
- WHO. (2023). Depression. World Health Organization. https://www.who.int/news-room/fact-sheets/detail/depression
- Wong, D. T., Bymaster, F. P., Horng, Y. S., & Molloy, B. B. (1995). The mechanism of action of sertraline is linked to potent blockade of serotonin uptake. Annals of the New York Academy of Sciences, 750(1), 380-392.
Be the first to comment