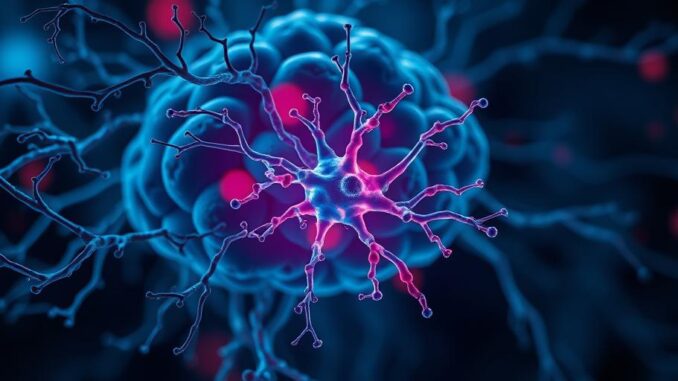
Summary
This article explores the groundbreaking use of machine learning in addiction research, focusing on how it reveals the dynamic role of astrocytes in heroin relapse. It also discusses the broader implications for addiction treatment and the potential for developing targeted therapies. The research offers hope for improved relapse prevention strategies and a deeper understanding of addiction’s complexities.
** Main Story**
Decoding Addiction: A Machine Learning Perspective
Substance use disorders? They’re a global problem, no question. We’ve made strides in understanding the neurobiological aspects of addiction through traditional research, but the brain is just so darn complex. That’s where innovative approaches, like machine learning, come in. And a recent study from the University of Cincinnati and the University of Houston? Well, it’s pretty exciting; potentially revolutionizing how we approach treatment and, crucially, relapse prevention.
The Astrocyte’s Role: Not Just a Supporting Actor
So, this research? It used object recognition software – think facial recognition or self-driving cars – to analyze changes in brain cell structure after heroin use, withdrawal, and relapse. Pretty cool, huh? You see, while previous research has largely focused on neurons, this study shines a light on astrocytes. What are they? Well they’re another type of brain cell, and it turns out they play a critical role.
For years, astrocytes were considered mere ‘support’ cells for neurons. But, it turns out they are dynamic players in synaptic activity. They do a ton! Provide metabolic support, regulate neurotransmitter levels, and even modulate synaptic plasticity. And the study? It revealed that astrocytes in the nucleus accumbens (NAc) – a key brain region for reward and motivation – undergo significant structural changes when exposed to heroin.
Machine Learning: Uncovering What We Couldn’t See Before
The researchers utilized machine learning algorithms to analyze images of astrocytes in the NAc of animal models. And these algorithms? Trained to recognize tiny differences in cell shape and size, they detected distinct patterns of astrocyte alterations following heroin use, withdrawal, and relapse. Specifically – and this is key – the study found that heroin exposure causes astrocytes to shrink and become less malleable. It’s thought this potentially hinders their ability to regulate synaptic activity and maintain homeostasis within the NAc. Imagine a stressed-out support system, unable to properly do its job. That’s kind of what’s happening here.
What Does This Mean for Relapse Prevention?
The implications are significant. These structural changes in astrocytes may contribute to the increased vulnerability to relapse seen in individuals struggling with heroin addiction. By disrupting the normal function of these cells, heroin potentially impairs the brain’s ability to adapt to the absence of the drug. And, as a result, it increases the likelihood of relapse when exposed to drug-related cues. Haven’t we all seen that? The trigger that sparks everything all over again?
This research? It opens new doors for developing targeted therapies. By focusing on restoring the normal structure and function of astrocytes, it might be possible to enhance relapse prevention strategies. Imagine a future where we can ‘tune up’ these brain cells to help people stay on the path to recovery. Moreover, the ability of machine learning to identify subtle cellular changes could also lead to personalized treatments. Treatments tailored to each individual’s specific neurobiological profile. Now that would be something, wouldn’t it?
Beyond Heroin: A Wider Lens
But the implications don’t stop with heroin. The application of machine learning to analyze cell structure can be applied to other substance use disorders, and even neurological conditions! By providing a deeper understanding of how different cell types contribute to disease, this technology can accelerate the development of more effective and targeted treatments for a wide array of ailments.
That said, there are things to be wary of! I remember a project I worked on last year that utilized very similar tech. While the results were fascinating, our team did have to deal with a large amount of image noise and bias. So its not perfect, but its a great tool!
The Future of Addiction Research: A Brighter Horizon
Frankly, this innovative application of machine learning is a huge step forward in addiction research. By uncovering the dynamic role of astrocytes and providing a powerful tool for analyzing cellular changes, this research has opened new doors for understanding and treating addiction. As machine learning techniques continue to evolve, they hold immense promise. They could further unravel the complexities of the brain and pave the way for more effective and personalized treatments for substance use disorders. It’s an exciting time, and I, for one, am eager to see what the future holds.
Be the first to comment