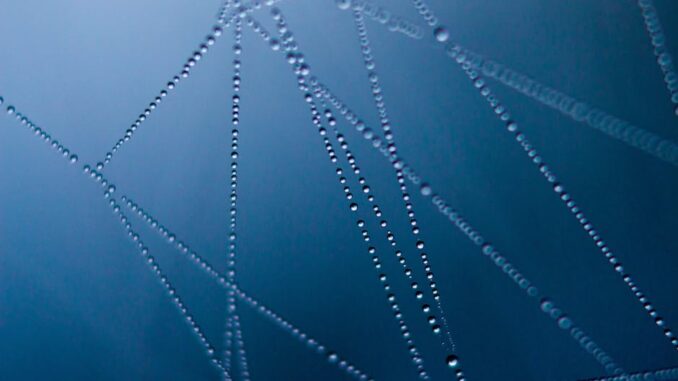
Abstract
Brain connectivity, encompassing structural, functional, and effective interactions within neural circuits, has emerged as a powerful tool for understanding complex cognitive and behavioral processes. While initial applications focused on mapping healthy brain organization and identifying disruptions in neurological disorders, the potential of connectomics to predict future behavioral outcomes is gaining increasing attention. This research report explores the current state of connectomics research as a predictive biomarker across a diverse range of domains, including, but not limited to, substance use, mental health, cognitive decline, and social behavior. We delve into the neuroimaging techniques employed to assess brain connectivity, discuss the different types of connectivity and their underlying mechanisms, and examine the neural networks implicated in various behavioral outcomes. Furthermore, we critically evaluate the methodological challenges, limitations, and ethical considerations associated with utilizing connectivity as a predictive marker. Finally, we propose future directions for research aimed at refining predictive models and translating connectomics findings into clinically relevant applications.
Many thanks to our sponsor Maggie who helped us prepare this research report.
1. Introduction
The human brain, a complex and dynamic network of interconnected neurons, is responsible for generating the myriad thoughts, emotions, and behaviors that define our individual experiences. Understanding the intricate relationships between different brain regions, and how these relationships give rise to emergent cognitive functions, is a central goal of neuroscience. Connectomics, the comprehensive mapping and analysis of neural connections, provides a powerful framework for investigating these relationships. Early connectomics research largely focused on describing the structural organization of the brain, employing techniques such as diffusion tensor imaging (DTI) to map white matter tracts. However, the field has rapidly expanded to encompass functional and effective connectivity, which capture the dynamic interactions between brain regions during task performance and at rest.
The allure of connectomics lies in its potential to move beyond simply describing brain organization to predicting future behavioral outcomes. The premise is that individual differences in brain connectivity reflect underlying variations in cognitive architecture and predispositions, making connectivity a valuable biomarker for predicting vulnerability to disease, response to treatment, or future behavioral choices. This predictive power has significant implications for personalized medicine, early intervention strategies, and a deeper understanding of the neurobiological mechanisms underlying complex human behavior.
While the potential of connectomics as a predictive biomarker is immense, significant challenges remain. The complexity of brain networks, the limitations of current neuroimaging techniques, and the influence of environmental factors all contribute to the difficulty of developing accurate and reliable predictive models. Moreover, ethical considerations regarding the use of predictive biomarkers, particularly in vulnerable populations, must be carefully addressed. This research report aims to provide a comprehensive overview of the current state of connectomics research as a predictive biomarker, highlighting both the exciting opportunities and the critical challenges that lie ahead.
Many thanks to our sponsor Maggie who helped us prepare this research report.
2. Neuroimaging Techniques for Assessing Brain Connectivity
A diverse array of neuroimaging techniques are employed to assess brain connectivity, each with its own strengths and limitations. These techniques can be broadly categorized based on their ability to measure structural, functional, or effective connectivity.
2.1 Structural Connectivity
Structural connectivity refers to the physical connections between brain regions, primarily mediated by white matter tracts. Diffusion tensor imaging (DTI) is the most widely used technique for mapping structural connectivity in vivo. DTI measures the diffusion of water molecules within the brain, which is constrained by the orientation of white matter fibers. By analyzing the diffusion patterns, researchers can reconstruct the trajectories of white matter tracts and quantify various properties of the white matter, such as fractional anisotropy (FA), which reflects the degree of directionality of diffusion.
Limitations of DTI include its sensitivity to crossing fibers, which can lead to inaccurate reconstruction of white matter tracts, and its inability to directly visualize individual axons. Advanced diffusion imaging techniques, such as diffusion spectrum imaging (DSI) and constrained spherical deconvolution (CSD), have been developed to address these limitations and provide more accurate estimates of structural connectivity.
2.2 Functional Connectivity
Functional connectivity refers to the statistical dependencies between the activity of different brain regions, irrespective of whether these dependencies are mediated by direct structural connections. Functional connectivity is typically assessed using functional magnetic resonance imaging (fMRI) or electroencephalography (EEG).
2.2.1 fMRI: fMRI measures brain activity by detecting changes in blood flow. Resting-state fMRI (rs-fMRI), in which participants are instructed to simply relax and not engage in any specific task, is a widely used method for assessing functional connectivity. During rs-fMRI, spontaneous fluctuations in brain activity occur, and the correlations between these fluctuations in different brain regions are interpreted as reflecting functional connectivity. Seed-based correlation analysis, independent component analysis (ICA), and graph theory are common methods for analyzing rs-fMRI data.
2.2.2 EEG: EEG measures electrical activity in the brain using electrodes placed on the scalp. EEG has excellent temporal resolution, allowing for the detection of rapid changes in brain activity. Functional connectivity in EEG can be assessed by analyzing the coherence or phase synchronization between EEG signals recorded from different electrodes. EEG-based connectivity measures are particularly useful for studying dynamic changes in brain connectivity during cognitive tasks or in response to stimuli.
Limitations of fMRI include its relatively poor temporal resolution (on the order of seconds) and its sensitivity to motion artifacts. EEG, while having excellent temporal resolution, suffers from poor spatial resolution due to the blurring of electrical signals as they pass through the skull.
2.3 Effective Connectivity
Effective connectivity refers to the influence that one brain region exerts over another. Unlike functional connectivity, which simply describes statistical dependencies, effective connectivity aims to infer causal relationships between brain regions. Dynamic causal modeling (DCM) and Granger causality are common methods for assessing effective connectivity. DCM involves specifying a generative model of how different brain regions interact and then estimating the parameters of the model based on fMRI data. Granger causality assesses whether the past activity of one brain region can predict the future activity of another region, suggesting a causal influence.
The interpretation of effective connectivity measures can be challenging, as they are based on model assumptions and can be sensitive to noise and artifacts in the data. Moreover, the causal inferences drawn from these measures are not necessarily equivalent to direct causal effects, as they may be mediated by unobserved variables.
Many thanks to our sponsor Maggie who helped us prepare this research report.
3. Neural Networks and Behavioral Outcomes: Predictive Applications
Numerous studies have demonstrated the potential of brain connectivity to predict future behavioral outcomes across a variety of domains. This section highlights some key examples.
3.1 Substance Use
Research has shown that functional connectivity patterns in specific brain networks can predict future substance use behavior. For example, altered connectivity within the reward system, including the ventral striatum and prefrontal cortex, has been associated with an increased risk of relapse in individuals with substance use disorders. Studies have also identified connectivity patterns that predict the likelihood of initiating substance use in adolescents. Furthermore, functional connectivity can differentiate between different substances of abuse and predict the severity of addiction.
Specific studies using resting-state fMRI have shown that reduced functional connectivity between the prefrontal cortex and the amygdala is associated with increased impulsivity, a known risk factor for substance use disorders. Similarly, increased connectivity within the default mode network (DMN), a network associated with self-referential thought, has been linked to craving and relapse in individuals with alcohol use disorder.
3.2 Mental Health
Brain connectivity has also shown promise as a predictive biomarker for mental health disorders. Studies have found that functional connectivity patterns can predict the development of depression, anxiety, and psychosis. For instance, altered connectivity within the salience network, which is involved in detecting and responding to salient stimuli, has been associated with an increased risk of developing psychosis. Reduced connectivity between the amygdala and the prefrontal cortex has been linked to increased anxiety and impaired emotion regulation.
Specifically, research on individuals at high risk for developing schizophrenia has shown that altered functional connectivity in the DMN and the frontoparietal network can predict the onset of psychotic symptoms. In addition, studies on patients with bipolar disorder have identified connectivity patterns that differentiate between manic and depressive states and predict the likelihood of future mood episodes.
3.3 Cognitive Decline
Connectomics has also been applied to predict cognitive decline and the progression of neurodegenerative diseases, such as Alzheimer’s disease. Studies have shown that disruptions in structural and functional connectivity can predict the rate of cognitive decline in individuals with mild cognitive impairment (MCI), a precursor to Alzheimer’s disease. Reduced connectivity in the hippocampus and other brain regions involved in memory has been associated with an increased risk of developing Alzheimer’s disease.
Longitudinal studies have demonstrated that structural connectivity measures, such as white matter integrity, can predict future cognitive performance in older adults. Furthermore, functional connectivity patterns can differentiate between individuals with stable MCI and those who will progress to Alzheimer’s disease.
3.4 Social Behavior
Emerging research suggests that brain connectivity can also predict social behavior, such as empathy, social cognition, and interpersonal interactions. Studies have found that connectivity within the social brain network, including the temporoparietal junction (TPJ) and the medial prefrontal cortex (mPFC), is associated with individual differences in empathy and social understanding. Altered connectivity in these regions has been linked to social deficits in individuals with autism spectrum disorder.
Specifically, research has shown that increased functional connectivity between the amygdala and the mPFC is associated with improved social cognition and the ability to accurately interpret social cues. Similarly, studies on individuals with high levels of psychopathy have found reduced connectivity between the amygdala and the ventromedial prefrontal cortex, which may contribute to their lack of empathy and remorse.
Many thanks to our sponsor Maggie who helped us prepare this research report.
4. Methodological Challenges and Limitations
Despite the promising potential of connectomics as a predictive biomarker, several methodological challenges and limitations must be addressed.
4.1 Data Acquisition and Processing
Neuroimaging data is inherently noisy and susceptible to artifacts, which can significantly impact the accuracy of connectivity estimates. Motion artifacts, physiological noise (e.g., cardiac and respiratory activity), and scanner-related artifacts must be carefully controlled for during data acquisition and preprocessing. Furthermore, the choice of preprocessing steps, such as spatial smoothing and temporal filtering, can influence the resulting connectivity patterns. Standardization of data acquisition and preprocessing protocols is crucial for ensuring the reproducibility and comparability of connectomics studies.
4.2 Statistical Analysis
Statistical analysis of connectomics data is computationally intensive and requires careful consideration of multiple comparisons. The number of possible connections in a brain network grows exponentially with the number of nodes, leading to a high risk of false positives. Appropriate statistical correction methods, such as false discovery rate (FDR) correction or family-wise error (FWE) correction, must be applied to control for multiple comparisons. Furthermore, the choice of statistical method, such as correlation analysis, regression analysis, or machine learning algorithms, can influence the results of the analysis.
4.3 Generalizability and Reproducibility
The generalizability and reproducibility of connectomics findings are major concerns. Many connectomics studies have small sample sizes, which limits the statistical power and increases the risk of overfitting the data. Furthermore, the results of connectomics studies can be highly dependent on the specific population studied, the imaging parameters used, and the analysis methods employed. Replication of connectomics findings in independent samples and across different research groups is essential for establishing the validity and reliability of connectivity-based biomarkers.
4.4 Causal Inference
While connectomics can identify associations between brain connectivity and behavioral outcomes, it is often difficult to infer causal relationships. Functional connectivity measures, in particular, are based on statistical dependencies and do not necessarily reflect direct causal influences. Effective connectivity methods, such as DCM and Granger causality, attempt to infer causal relationships, but they are based on model assumptions and can be sensitive to noise and artifacts in the data. Establishing causal relationships between brain connectivity and behavior requires the use of experimental manipulations, such as transcranial magnetic stimulation (TMS) or lesion studies.
Many thanks to our sponsor Maggie who helped us prepare this research report.
5. Ethical Considerations
The use of connectomics as a predictive biomarker raises several ethical considerations. The potential for predicting future behavioral outcomes raises concerns about privacy, discrimination, and self-fulfilling prophecies. If connectivity-based biomarkers are used to predict an individual’s risk of developing a mental health disorder or engaging in substance use, this information could be used to discriminate against them in employment, insurance, or other areas of life. Furthermore, if individuals are labeled as being at high risk for a particular outcome based on their brain connectivity, this could lead to self-fulfilling prophecies, where their behavior is influenced by the label itself.
It is crucial to ensure that the use of connectomics as a predictive biomarker is guided by ethical principles of fairness, transparency, and respect for individual autonomy. Individuals should have the right to access and control their own brain connectivity data and to make informed decisions about whether or not to participate in connectomics research. Furthermore, safeguards must be put in place to prevent the misuse of connectivity-based biomarkers for discriminatory purposes.
Many thanks to our sponsor Maggie who helped us prepare this research report.
6. Future Directions
The field of connectomics as a predictive biomarker is rapidly evolving, and several promising avenues for future research exist.
6.1 Multimodal Imaging
Combining different neuroimaging techniques, such as fMRI and EEG, can provide a more comprehensive assessment of brain connectivity and improve the accuracy of predictive models. Multimodal imaging can leverage the strengths of each technique to overcome their individual limitations. For example, combining fMRI with its high spatial resolution and EEG with its high temporal resolution can provide a more detailed picture of the spatiotemporal dynamics of brain networks.
6.2 Longitudinal Studies
Longitudinal studies that track changes in brain connectivity and behavior over time are essential for understanding the developmental trajectories of brain networks and for identifying early predictors of behavioral outcomes. Longitudinal studies can also help to disentangle the effects of environmental factors and genetic influences on brain connectivity.
6.3 Machine Learning
Machine learning algorithms can be used to develop more sophisticated predictive models based on brain connectivity data. Machine learning can identify complex patterns in connectivity data that may not be apparent using traditional statistical methods. Furthermore, machine learning can be used to personalize predictive models by taking into account individual differences in brain connectivity and other factors.
6.4 Translational Applications
The ultimate goal of connectomics research is to translate findings into clinically relevant applications. Connectivity-based biomarkers could be used to identify individuals at high risk for developing mental health disorders or substance use disorders, allowing for early intervention strategies. Connectivity-based biomarkers could also be used to personalize treatment approaches by predicting an individual’s response to different therapies.
Many thanks to our sponsor Maggie who helped us prepare this research report.
7. Conclusion
Connectomics has emerged as a powerful tool for understanding the complex relationships between brain connectivity and behavior. While the potential of connectomics as a predictive biomarker is immense, significant challenges remain. Methodological limitations, ethical considerations, and the need for translational applications must be carefully addressed. By overcoming these challenges, connectomics can pave the way for personalized medicine, early intervention strategies, and a deeper understanding of the neurobiological mechanisms underlying complex human behavior. The integration of multimodal imaging, longitudinal study designs, and advanced machine learning techniques will be crucial for advancing the field and realizing the full potential of connectomics as a predictive biomarker.
Many thanks to our sponsor Maggie who helped us prepare this research report.
References
- Biswal, B. B., Yetkin, F. Z., Haughton, V. M., & Hyde, J. S. (1995). Functional connectivity in the motor cortex of resting human brain using echo-planar MRI. Magnetic Resonance in Medicine, 34(4), 537-541.
- Friston, K. J., Harrison, L., & Penny, W. (2003). Dynamic causal modelling. NeuroImage, 19(4), 1273-1302.
- Greicius, M. D., Srivastava, G., Reiss, A. L., & Menon, V. (2004). Default-mode network activity distinguishes Alzheimer’s disease from healthy aging: evidence from functional MRI. Proceedings of the National Academy of Sciences, 101(13), 4637-4642.
- Hagmann, P., Cammoun, L., Gigandet, X., Meuli, R., Honey, C. J., Wedeen, V. J., & Sporns, O. (2008). Mapping the structural core of human cerebral cortex. PLoS Biology, 6(7), e159.
- Honey, C. J., Sporns, O., Cammoun, L., Gigandet, X., Thiran, J. P., Meuli, R., & Hagmann, P. (2009). Predicting human resting-state functional connectivity from structural connectivity. Proceedings of the National Academy of Sciences, 106(6), 2035-2040.
- Raichle, M. E., MacLeod, A. M., Snyder, A. Z., Powers, W. J., Gusnard, D. A., & Shulman, G. L. (2001). A default mode of brain function. Proceedings of the National Academy of Sciences, 98(2), 676-682.
- Rubinov, M., & Sporns, O. (2010). Complex network measures for brain connectivity analysis: definitions and examples. NeuroImage, 52(3), 1059-1069.
- Smith, S. M., Fox, P. T., Miller, K. L., Glahn, D. C., Fox, P. M., Mackay, C. E., … & Beckmann, C. F. (2009). Correspondence of the brain’s functional architecture during activation and rest. Proceedings of the National Academy of Sciences, 106(31), 13040-13045.
- Sporns, O. (2011). Networks of the brain. MIT press.
- Van den Heuvel, M. P., & Hulshoff Pol, H. E. (2010). Exploring the brain network: a review on resting-state fMRI functional connectivity. European Neuropsychopharmacology, 20(8), 519-534.
Be the first to comment